QuSmart® Tech Explainer
AI-Native Perfect Secrecy for Single Frequency Network (SFN) Security in ATSC 3.0 BPS
QuSmart.AI ensures that SFNs remain secure, adaptive, and future-proof against evolving cyber threats.
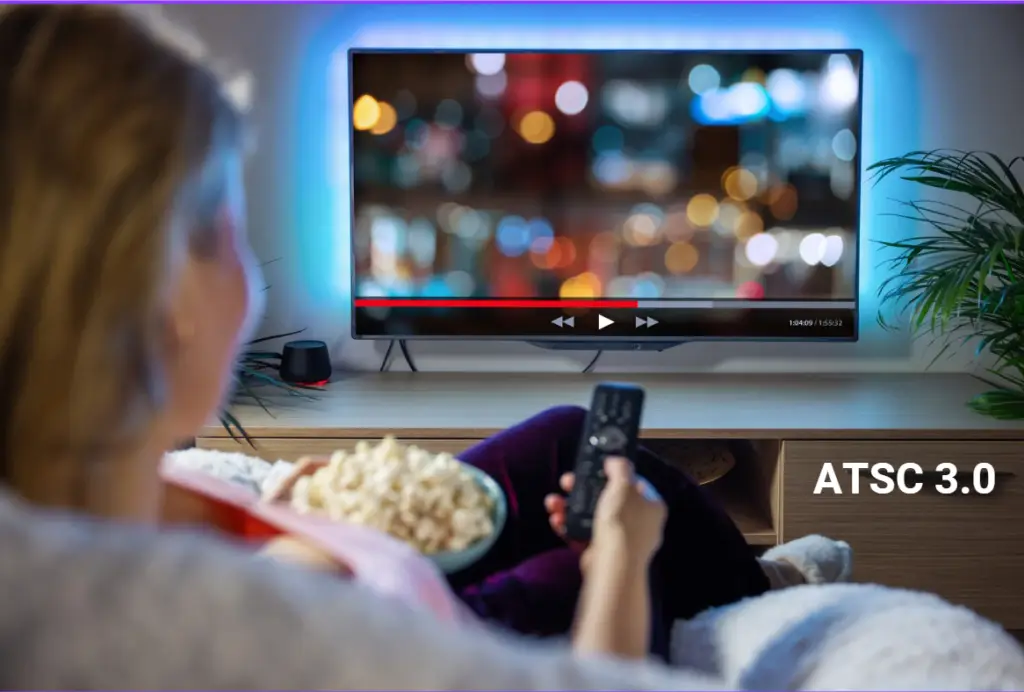
Tracy Levine, QuSmart.AI Co-Founder, CEO/CAIO
Cryptography Patent Writer
Abstract
As ATSC 3.0’s Single Frequency Networks (SFNs) expand to support precise time synchronization and Broadcast Positioning System (BPS) capabilities, security and resilience become critical. Traditional cryptographic models introduce latency, require complex key management, and remain vulnerable to quantum and AI-driven threats. QuSmart.AI’s AI-Native Shannon’s Perfect Secrecy (SPS) encryption offers a provable quantum-secure and AI-resistant solution for SFN time synchronization, signal authentication, and positioning security. This paper explores the technical integration of QuSmart.AI’s AI-driven encryption within SFN deployments, eliminating security risks while ensuring uninterrupted, high-precision ATSC 3.0 operations.
1. Introduction
1.1 The Security Challenges in SFN-Based BPS
- SFNs rely on precise time synchronization across multiple transmitters to avoid interference.
- GPS/GNSS dependency introduces jamming and spoofing vulnerabilities.
- Traditional cryptographic models require key distribution, creating potential attack vectors.
- AI-enhanced attacks can manipulate timing, desynchronize SFNs, or inject false positioning data.
1.2 QuSmart.AI’s AI-Native Perfect Secrecy Encryption
- AI is natively embedded into the encryption, eliminating predictable attack surfaces.
- No stored, distributed, or exchanged keys—ensuring zero key exposure.
- Real-time adaptive encryption provides self-healing, autonomous SFN synchronization.
- Quantum-immune encryption protects against both AI and quantum computing threats
2. AI-Native Encryption for SFN Time Synchronization
2.1 Eliminating GPS Dependency with AI-Encrypted Mesh Timing
✅ Decentralized synchronization: QuSmart.AI’s AI-managed encryption enables SFN nodes to share time signals securely without relying on GNSS.
✅ No key management overhead: Unlike TLS or PQC-based methods, Perfect Secrecy encryption eliminates key storage or distribution risks.
✅ Resilient against jamming and spoofing: Encrypted timing packets remain statistically independent, making them impossible to forge.
Implementation Steps:
- Leader Transmitters Establish Encrypted Time Reference
- Leader SFN nodes generate encrypted time synchronization packets using AI-driven SPS.
- This ensures real-time clock distribution without external dependencies.
- Mesh-Based SFN Synchronization
- Follower SFN nodes receive encrypted time packets from multiple neighbors.
- AI-based self-correction dynamically adjusts node timing to prevent drift.
- Adaptive Time Drift Correction
- AI continuously monitors and adjusts time synchronization across the SFN mesh.
- Even if some nodes fail, real-time self-healing synchronization maintains network integrity
3. PIN-Based Authentication for SFN Messaging
3.1 Preventing Unauthorized Transmitters in SFNs
✅ No shared secrets or certificates: Shannon’s Perfect Secrecy PIN-based authentication eliminates reliance on traditional key-based authentication.
✅ Statistically unique PIN-encrypted messages: Prevents replay attacks or injection of rogue synchronization data.
✅ Real-time classification of encrypted data: AI dynamically processes and categorizes SFN communications based on PIN-tagging.
Implementation Steps:
- Assign Unique PINs for SFN Nodes
- Each SFN node receives a unique AI-managed PIN to categorize time synchronization data.
- Example PIN-based categories: CONFIRMED, NOT-CONFIRMED, OBSERVATION, FAKE, etc.
- AI-Driven Secure Messaging Processing
- AI validates PIN-tagged SFN messages before synchronization updates are applied.
- Only statistically valid encrypted data is processed, rejecting potential threats.
- Automated Detection & Blocking of Rogue Transmitters
- If an SFN node detects an unauthorized transmission, AI flags it network-wide.
- Other nodes automatically block and ignore unauthorized signals.
4. Transcryption for Secure SFN-BPS Signal Integrity
4.1 Protecting SFN-Based Positioning Data
✅ Unified encryption for control and data planes: Prevents adversaries from manipulating BPS metadata.
✅ Eliminates multipath interference vulnerabilities: Encrypted waveforms mitigate false TOA calculations.
✅ Ensures only authentic position data is processed: AI-driven authentication rejects manipulated location signals.
Implementation Steps:
- QuSmart TransCryption of BPS Metadata and Positioning Data
- All BPS messages, including TOA timestamps, undergo real-time AI-driven Perfect Secrecy encryption.
- Only properly encrypted transmissions contribute to location calculations.
- Adaptive Filtering of Multipath Reflections
- AI-based filtering separates valid SFN signals from reflected/multipath artifacts.
- Only direct line-of-sight TOA values are used for positioning computations.
- Quantum-Resistant SFN Navigation Systems
- Autonomous vehicle navigation, military positioning, and emergency response applications benefit from fully quantum-secure SFN-based BPS.
5. AI-Driven Flexible I/O for SFN Integration
5.1 Seamless Encrypted Data Flow Across SFN Nodes
✅ Ensures compatibility with ATSC 3.0 infrastructure: AI-managed encryption seamlessly integrates with legacy and modern broadcast networks.
✅ Prevents cyber threats through continuous monitoring: AI dynamically adjusts encryption without requiring manual intervention.
✅ Self-healing security mechanisms: If an SFN link is disrupted, AI automatically re-establishes a secure channel.
Implementation Steps:
- Configure AI-Managed Flexible I/O
- SFN transmitters and receivers operate within an AI-driven encrypted environment.
- No external key exchange or complex configurations required.
- Dynamic Real-Time Threat Detection
- AI continuously monitors encrypted SFN transmissions for potential cyber threats.
- If an anomaly is detected, the encryption dynamically adapts to neutralize the attack.
- Self-Healing Network Encryption
- In case of connectivity disruption, AI instantly establishes a new secure transmission path.
- This eliminates downtime or potential attack vectors that could exploit broken connections.
Why QuSmart.AI’s AI-Native Perfect Secrecy is the Future of SFN Security
SFN Security Issue | How QuSmart.AI’s AI-Native SPS Solves It |
GNSS dependency for SFN synchronization | AI-encrypted mesh timing eliminates GNSS risks |
Key management complexity | No key storage, transmission, or distribution |
Multipath interference in SFN-BPS | AI-driven encrypted waveforms filter reflections |
Rogue SFN transmitter attacks | PIN-based authentication prevents spoofing |
Manual security configurations | AI-driven Flexible IO enables self-healing encryption |
WIN:
🔹 By leveraging AI-Native Perfect Secrecy, ATSC 3.0 SFNs achieve self-sustaining, quantum-immune, and AI-resilient encryption, eliminating security weaknesses associated with legacy cryptographic approaches.
*Patent Pending Technology